Note
Click here to download the full example code
UoI-NMF for robust parts-based decomposition of noisy data
This example will demonstrate parts-based decomposition with UoI-NMF on the swimmer dataset. The swimmer dataset is the canonical example of separable data.
Swimmer dataset
import matplotlib
import matplotlib.pyplot as plt
import numpy as np
from sklearn.preprocessing import minmax_scale
from sklearn.manifold import TSNE
from pyuoi.decomposition import UoI_NMF
from pyuoi.datasets import load_swimmer
matplotlib.rcParams['figure.figsize'] = [4, 4]
np.random.seed(10)
swimmers = load_swimmer()
swimmers = minmax_scale(swimmers, axis=1)
Original Swimmer samples
fig, ax = plt.subplots(4, 4, subplot_kw={'xticks': [], 'yticks': []})
indices = np.random.randint(16, size=16) + np.arange(0, 256, 16)
ax = ax.flatten()
for i in range(len(indices)):
ax[i].imshow(swimmers[indices[i]].reshape(32, 32).T,
aspect='auto', cmap='gray')
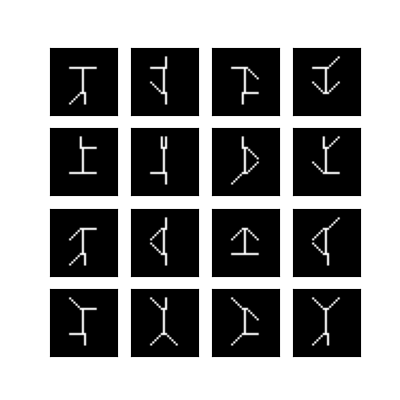
Swimmer samples corrupted with Absolute Gaussian noise
Corrupt the images with with absolute Gaussian noise with std = 0.25
.
reps = 1
n_swim = swimmers.shape[0]
corrupted = np.zeros((n_swim * reps, swimmers.shape[1]))
for r in range(reps):
noise = np.abs(np.random.normal(scale=0.25, size=swimmers.shape))
corrupted[r * n_swim:(r + 1) * n_swim] = swimmers + noise
fig, ax = plt.subplots(4, 4, subplot_kw={'xticks': [], 'yticks': []})
ax = ax.flatten()
for i in range(len(indices)):
ax[i].imshow(corrupted[indices[i]].reshape(32, 32).T,
aspect='auto', cmap='gray')
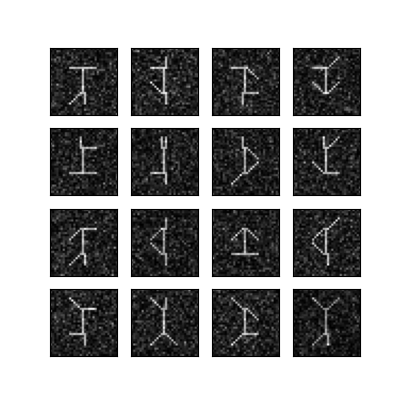
Run UoI NMF on corrupted Swimmer data
Twenty bootstraps should be enough.
min_pts
should be half of the number of bootstraps.
nboot = 20
min_pts = nboot / 2
ranks = [16]
shape = corrupted.shape
uoi_nmf = UoI_NMF(n_boots=nboot, ranks=ranks, db_min_samples=min_pts,
nmf_max_iter=800)
transformed = uoi_nmf.fit_transform(corrupted)
recovered = transformed @ uoi_nmf.components_
NMF Swimmer bases
order = np.argsort(np.sum(uoi_nmf.components_, axis=1))
fig, ax = plt.subplots(4, 4, subplot_kw={'xticks': [], 'yticks': []})
ax = ax.flatten()
for i in range(uoi_nmf.components_.shape[0]):
ax[i].imshow(uoi_nmf.components_[order[i]].reshape(32, 32).T,
aspect='auto', cmap='gray')
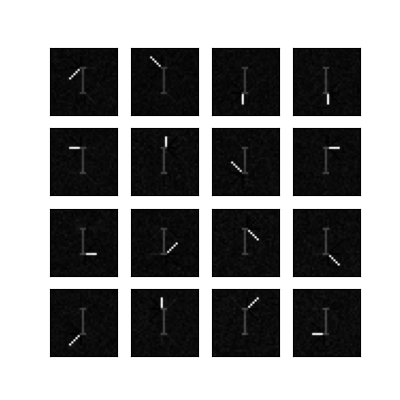
Recovered Swimmers
fig, ax = plt.subplots(4, 4, subplot_kw={'xticks': [], 'yticks': []})
ax = ax.flatten()
for i in range(len(indices)):
ax[i].imshow(recovered[indices[i]].reshape(32, 32).T,
aspect='auto', cmap='gray')
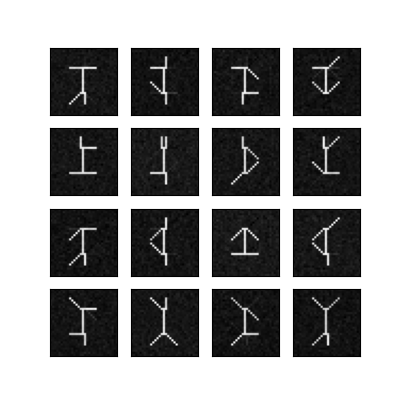
Plot them all together so we can see how well we recovered the original swimmer data.
fig, ax = plt.subplots(3, 16, figsize=(27, 5),
subplot_kw={'xticks': [], 'yticks': []})
indices = np.random.randint(16, size=16) + np.arange(0, 256, 16)
ax = ax.flatten()
# plot Original
ax[0].set_ylabel('Original', rotation=0, fontsize=25, labelpad=40)
ax[0].yaxis.set_label_coords(-1.0, 0.5)
for i in range(len(indices)):
ax[i].imshow(swimmers[indices[i]].reshape(32, 32).T,
aspect='auto', cmap='gray')
# plot Corrupted
ax[16].set_ylabel('Corrupted', rotation=0, fontsize=25, labelpad=40)
ax[16].yaxis.set_label_coords(-1.1, 0.5)
for i in range(len(indices)):
ax[16 + i].imshow(corrupted[indices[i]].reshape(32, 32).T,
aspect='auto', cmap='gray')
# plot Recovered
ax[32].set_ylabel('Recovered', rotation=0, fontsize=25, labelpad=40)
ax[32].yaxis.set_label_coords(-1.1, 0.5)
for i in range(len(indices)):
ax[32 + i].imshow(recovered[indices[i]].reshape(32, 32).T,
aspect='auto', cmap='gray')

To see what DBSCAN is doing, let’s look at the bases samples.
plt.figure()
embedding = TSNE(n_components=2).fit_transform(uoi_nmf.bases_samples_)
sc = plt.scatter(embedding[:, 0], embedding[:, 1],
c=uoi_nmf.bases_samples_labels_, s=80, cmap="nipy_spectral")
sc.set_facecolor('none')
plt.show()
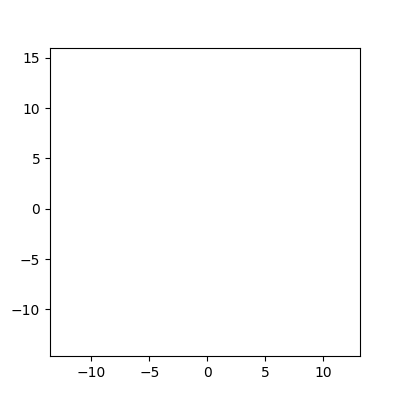
Out:
/home/docs/checkouts/readthedocs.org/user_builds/pyuoi/envs/latest/lib/python3.7/site-packages/sklearn/manifold/_t_sne.py:783: FutureWarning: The default initialization in TSNE will change from 'random' to 'pca' in 1.2.
FutureWarning,
/home/docs/checkouts/readthedocs.org/user_builds/pyuoi/envs/latest/lib/python3.7/site-packages/sklearn/manifold/_t_sne.py:793: FutureWarning: The default learning rate in TSNE will change from 200.0 to 'auto' in 1.2.
FutureWarning,
Total running time of the script: ( 0 minutes 41.427 seconds)